Machine Learning- Deep Learning for Advanced Analytics
- Masoud Nikravesh
- Nov 22, 2015
- 2 min read

Deep Learning is a step towards realizing strong Artificial Intelligence. While, Deep Learning is rebranding of Neural Network which goes back to 1980s and even 1960s, many organizations including Google, Facebook, Microsoft, Baidu, among others showed interest in its use for specific applications. Facebook hired Yann LeCun to head its artificial intelligence and later hired Vladimir Vapnik the co-inventor of the support vector machine method. Google hired Geoffrey Hinton to focus on improving machine learning and deal with the growing amount of its data. Microsoft established the Deep Learning Technology Center while Baidu hired Andrew Ng to head its Silicon Valley-based research lab focusing on deep learning.

We use state-of-the art Neuro Computing and Deep Learning architectures such as deep neural networks, deep Boltzmann and Restricted Boltzmann machine, convolutional deep neural networks, deep belief networks and recurrent neural networks for advanced analytics, fraud detection, anomaly and novelty detection, medical diagnosis and prognosis, classification, and clustering.
The current success of neural networks and rebranding to deep learning neural network is due in part to the availability and easy access to massive increase in [Cheap] computing power, [Cheap] large scale memory, [Cheap] cloud computing infrastructure, [Cheap] storage and massive increase in the amount of available interesting real world data sets with high-payoff business opportunities. Some of these deep neural networks models are composed of hundreds of millions of parameters/weights and millions of nodes that can be built, and rapidly train using large interesting real world data sets, and can quickly deployed into production across a wide variety of different platforms such as clouds, phones, sensors, etc.
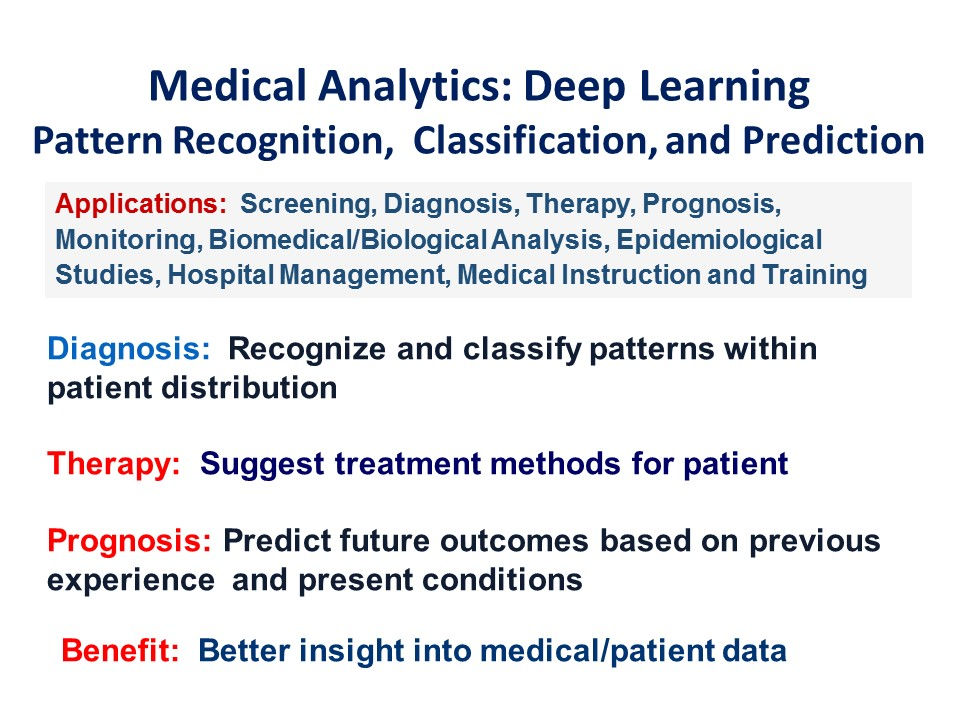
Our technology go beyond the traditional method and replay on Artificial Intelligence/Machine Learning-deep learning technologies and advanced analytics utterly embedded and complemented with advanced platform that can extract actionable insights at speed and scale. We model user’s behavior at scale based on evolutionary models to create all the possible patterns and users’s behavior. We use Artificial Intelligence and Deep Learning technologies combined with evolutionary behavioral predictive, graph and advanced analytics to no learn from the past user activities, and behavior, and provide actionable insight. Our technology can also monitor, identify, classify, and predict, abnormal and/or suspicious user activity and behavior, including possible frauds. The system can also predict anomaly and novelty as it happens and as it develops in real time and provide real time alert/notification, and actionable insights. Some of deep neural networks models that we have developed have over hundreds of millions of weights and hundreds of thousands to millions of nodes. These models can be be built either using cloud services or in house computing infrastructures. They can rapidly be trained using large interesting real world data sets, and can be quickly deployed into production across a wide variety of different platforms.
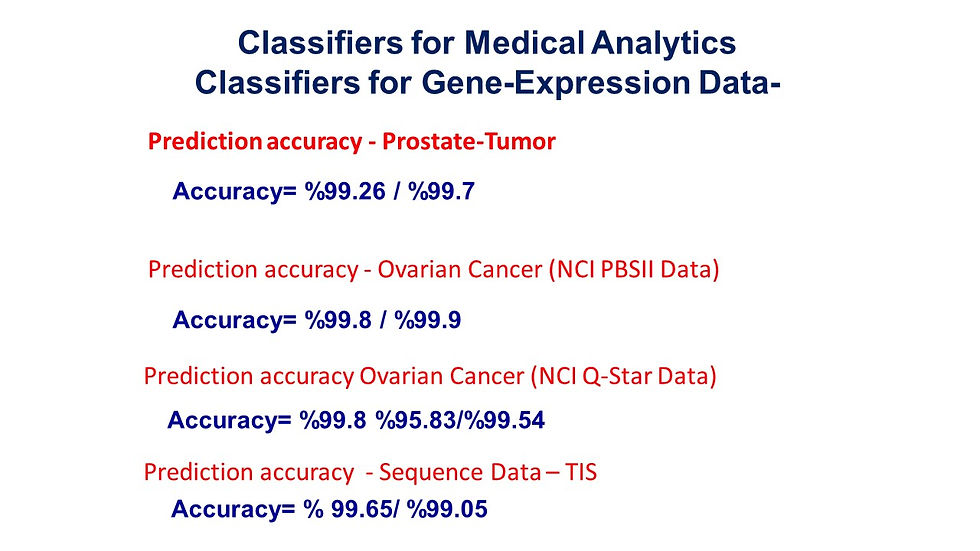
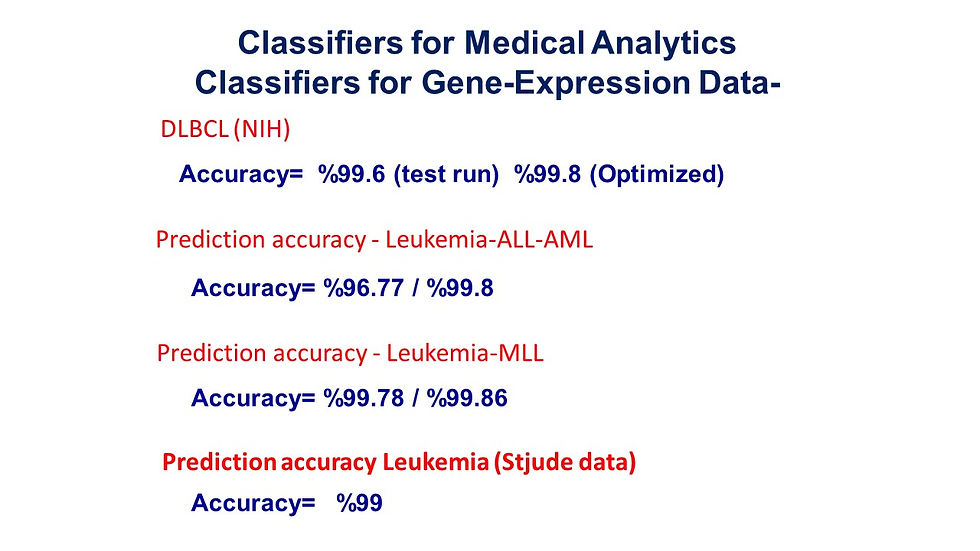
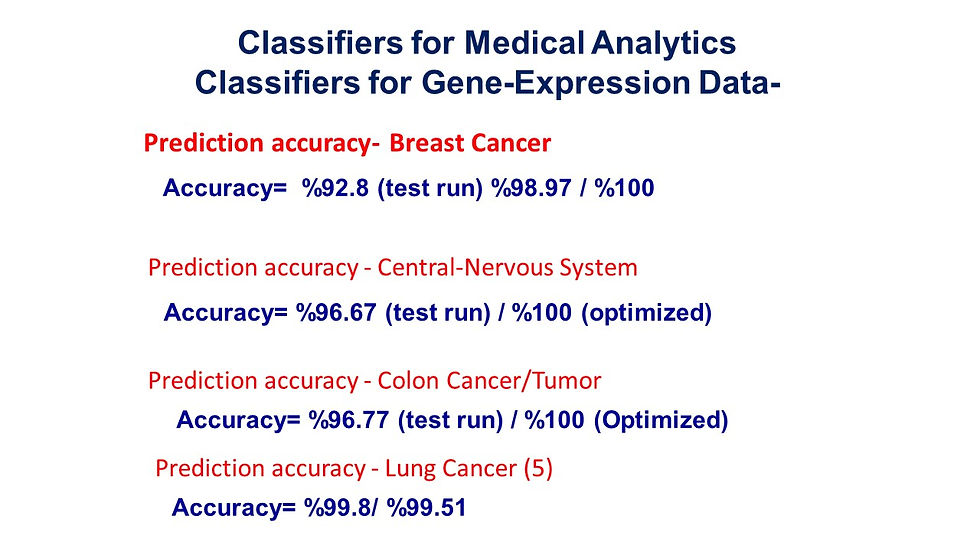
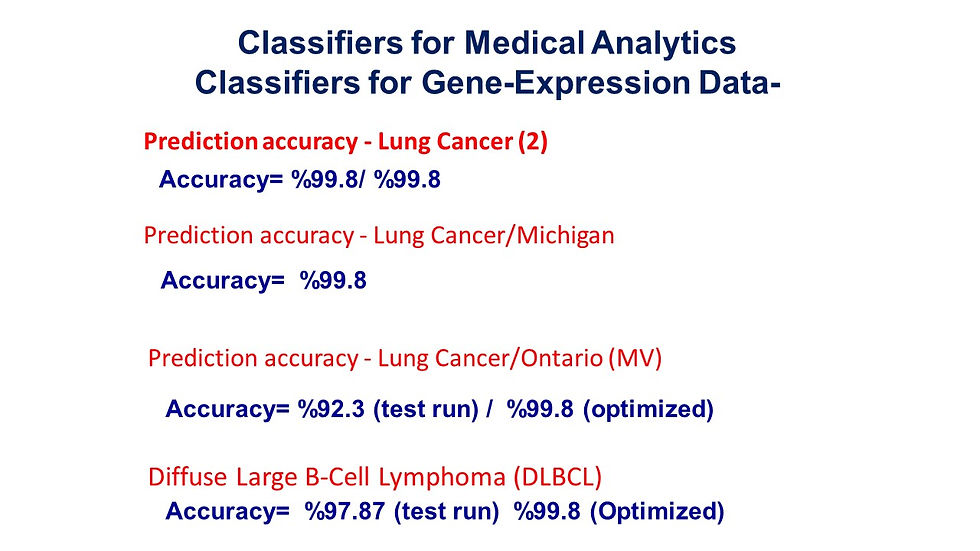
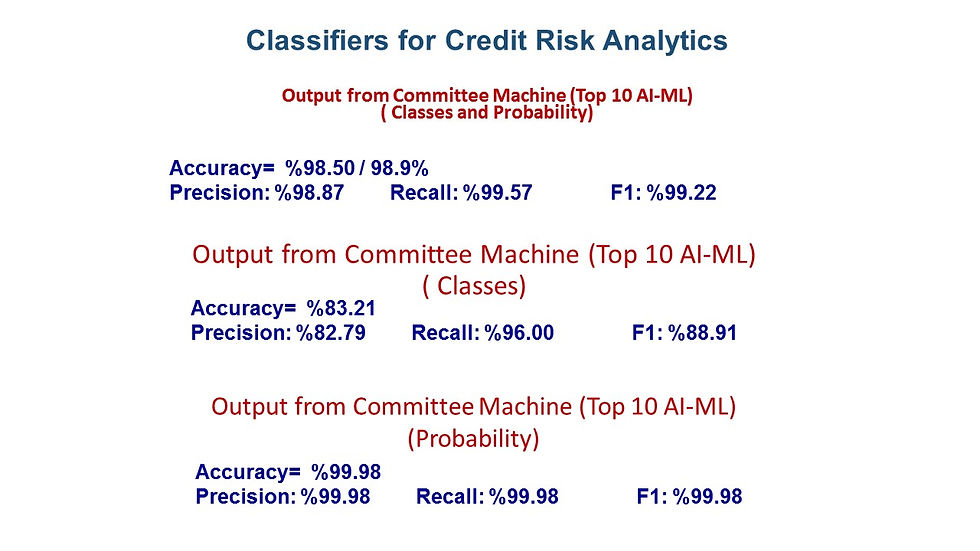
Comments